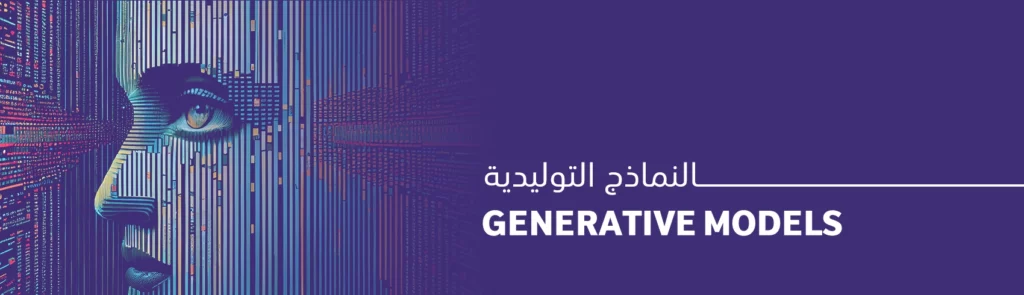
Generative Models
With the rapid developments of technology and artificial intelligence in recent times, many applications have emerged that generate text, images, and videos based on text entered by the user. These applications have revolutionized various fields from computer vision and natural language processing to art and design. The engine behind these applications is generative models. In this article we will take a look at generative models, their applications and the possibilities they offer.
What are generative models?
Generative models are a type of artificial intelligence model that is used to create and generate new data that is similar to the original data on which it was trained. These models aim to discover the basic patterns and structures of the original data, so as to be able to create new data similar to the original data. [1]
What are the applications of generative models?
Generative models have become increasingly popular recently for their ability to generate more realistic and accurate data whether it is images, videos, texts, etc.
It has become used in many applications such as image and video synthesis, text generation, and even drug discovery.
They can be used in photo and video montage to create more diverse and realistic images and videos. Which can be used in virtual reality applications and games, as well as in various designs such as interior design and user interface design.
It can also be used to create and generate texts such as writing emails, writing a blog article or a social media post.
In the field of drug discovery, generative models can be used to generate new drug compounds, especially when new and rapidly spreading epidemics emerge, such as COVID-19, as generative models have been used to generate the computational design of therapeutic molecules against the COVID-19 virus by training them on a large set of drug data. And antibodies to similar viruses. [2]
How do generative models work?
There is a large group of generative models, each of which has its own method, but we can review how one of these models works to get an idea of the way these models can generate new, more realistic data.
A Generative Adversarial Network (GAN) is a generative network consisting of two neural networks: a generative network and a recognition network, as shown in the figure below.
The generative network is trained to produce samples of data similar to the original data, while the discriminator network is trained to differentiate between the original data and the generated data.
During training, generative networks are trained and discriminated against each other. The generative network attempts to produce samples of data that can fool the discriminator network into believing it is real, while the discriminator network attempts to correctly identify true and fake samples.
Over time, the generative network becomes better at producing realistic samples of data, while the discriminator network becomes better at distinguishing between real and fake samples. Eventually, the generative network learns to produce samples of data that are almost indistinguishable from the original data. [1]

What are the challenges of generative modeling?
There are many challenges facing generative models, the most important of which are the following:
Computational requirements: Generative AI systems often need a large amount of data and computational power, and this can be very expensive and time-consuming.
Quality of generated output: The output generated by generative models may not be completely accurate or error-free. This is due to a number of reasons, including lack of data, insufficient training, or the model being too complex. [3]
What are the risks of generative models?
Every technology is a double-edged sword. Just as it has many advantages, there are some potential risks to generative models:
Information bias: Output from generative models may be biased toward a particular class, age, or race. Information bias often occurs when the training data set is limited and lacks sufficient data samples to adequately represent all possible input data values.
Information security: Generative AI systems can be used to spread false information or propaganda by creating fake videos, images and text, but they appear more realistic and convincing.
These risks can be overcome or their impact reduced by:
Develop national and international regulations to limit the incorrect use of this technology.
Establishing regulatory standards for the application of artificial intelligence in organizations and companies.
Make artificial intelligence part of the organization’s culture and discussions. The more awareness of this technology increases, the more individuals can avoid its risks.
Technology training with humanities perspectives.
Trends and future implications of generative models:
Generative models are considered one of the most important technologies that have influenced and will influence various fields such as customer service, marketing and sales, software engineering, research and development, as well as improving healthcare applications and other fields [5]. These effects are expected to lead to improved business and interaction between users and technology and to make interaction with computer systems easier and more streamlined. Here are some future trends and implications of generative models:
Generative models will help drive the creation of natural language interfaces that are more intuitive and easy to use, allowing users to communicate with computer systems using natural language rather than programming languages.
Enhance productivity and improve many repetitive daily tasks: Generative models have the ability to automate most of the work activities which occupy 60%-70% of an employee’s time. [5]
Improving health services: Generative models can improve patient outcomes by extracting and coding medical documentation, organizing medical, lifestyle and genetic information and improving patient engagement through personalized recommendations, medication reminders and symptom tracking.
Develop plans and strategies based on synthetic data: Generative models can improve the ability to simulate events, generate synthetic data, and identify the most effective scenarios. [4]
Increasing the economic return for companies, institutions, and entities: According to a report issued by McKinsey, generative artificial intelligence is expected to add $2.6 trillion to $4.4 trillion to the global economy annually. [5]
Generative modeling has become a driving force behind the creation of numerous applications and innovations, from creating photorealistic images and text to aiding in medical inventions and improving healthcare, as well as actively contributing to customer service and marketing. With the rapid advancement of this technology, consideration must be given to embracing the capabilities it provides, and learning the necessary skills to use it while taking into account ethical considerations and responsible use.
“Striking a balance between high-tech innovation and human-centered thinking is an ideal way to produce responsible technology and ensure the future of AI remains hopeful for the next generation.” Mike Thomas. [6]
“Balancing high-tech innovation with human-centered thinking is an ideal method for producing responsible technology and ensuring the future of AI remains hopeful for the next generation.” Mike Thomas [6]
the reviewer:
[1]: Deep Generative Models | by Prakash Pandey | Towards Data Science
[2]: Using Generative AI to Accelerate Drug Discovery | IBM Research Blog
[3]: What is Generative Modeling? |Definition from TechTarget
[4]: Generative AI landscape: Potential future trends | TechTarget
[5]: Economic potential of generative AI | McKinsey
[6]: 8 Risks and Dangers of Artificial Intelligence to Know | Built In
writing:
Naglaa Saud Al-Saedi
Artificial intelligence engineer